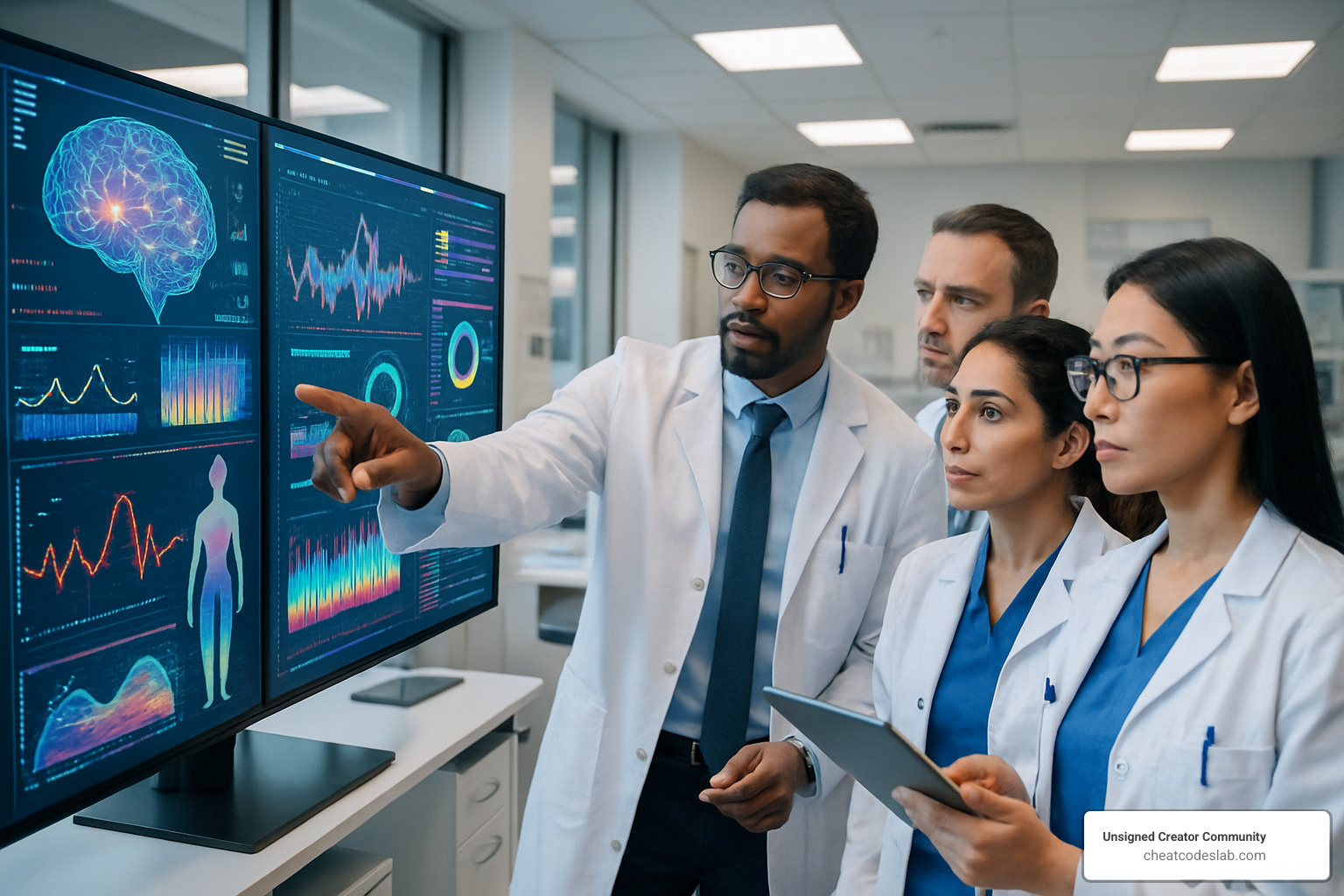
The AI Revolution in Medical Research: Changing How We Find Cures
Remember the last time you heard about a groundbreaking medical findy? Chances are, AI tools for medical research played a crucial role behind the scenes. As someone who’s witnessed this change firsthand, I’m excited to share how these innovations are reshaping the quest for medical breakthroughs.
The numbers tell a compelling story: bringing just one medication to market now costs over $1 billion and often takes more than a decade. Traditional research methods are buckling under these pressures, creating bottlenecks that slow progress when we need it most. From drowning in scientific literature to the painstaking process of recruiting clinical trial participants, researchers face problems at every turn.
This challenging landscape has led to what experts call “Eroom’s Law” – that’s Moore’s Law spelled backward. It describes a troubling trend where the number of drugs approved per billion dollars spent on R&D has halved approximately every nine years for six decades. But there’s good news on the horizon!
AI tools for medical research are breaking this cycle by changing how scientists work. Literature reviews that once took weeks now happen in days. Patient screening for clinical trials has shrunk from weeks to minutes. Diagnostic accuracy has reached new heights, and administrative paperwork is becoming less of a burden.
Consider what’s happening in different research areas:
For literature reviews, tools like Consensus, Elicit, and Rayyan give researchers instant access to over 200 million scientific papers, making reviews up to 50% faster. In clinical trials, platforms like Deep 6 AI, Saama, and Medidata are revolutionizing patient recruitment. Diagnostic imaging benefits from Google Health AI and MedLM, which can match radiologist accuracy while providing millions of free screenings. Meanwhile, drug findy tools like Causaly and Iris.ai are helping researchers overcome declining R&D productivity.
As a technology entrepreneur who’s collaborated with medical researchers for years, I’ve seen how these tools transform workflows and accelerate findies that save lives. My work has focused on helping clinical research professionals understand and adopt these powerful AI solutions – making complex technology accessible to those who need it most.
The beauty of AI tools for medical research isn’t just in their impressive capabilities, but in how they empower human researchers to do what they do best: ask creative questions, design innovative studies, and interpret results with the nuance that only human intelligence can provide.
Want to explore more about how AI is changing different fields? Check out these related resources:
The medical research landscape is changing rapidly, and those who accept these AI-powered approaches will lead the way toward faster, more affordable, and more effective healthcare solutions for all of us.
AI tools for medical research: Workflow-Based Tour
Ever wonder how researchers manage to sift through mountains of medical data? The research process is a winding road, full of twists, turns, and (let’s be honest) frustrating bottlenecks. Thankfully, AI tools for medical research are smoothing out these bumps, making each step of the journey more efficient and insightful.
Let’s take a guided tour through the research workflow to see how AI is changing each stage – from those first literature searches to the final implementation of life-saving treatments.
Literature Search & Review with AI tools for medical research
Remember the last time you tried to keep up with your field’s latest research? Overwhelming, right? With over 2 million new medical papers published each year, even the most dedicated researcher can’t possibly read everything relevant.
This is where tools like Consensus AI are changing the game. Imagine having instant access to over 200 million research papers with an AI that extracts key findings for you. Rather than skimming dozens of abstracts, you get direct answers to your scientific questions.
Michael Nielsen, PhD, puts it perfectly: “It’s rapidly surfaced a lot of interesting things I hadn’t found through traditional search engines.” The tool uncovers hidden connections that might take weeks to find manually.
For those marathon literature review sessions, Elicit helps researchers work at what they call “superhuman speed.” About 10% of users save 5+ hours weekly on literature tasks alone. The platform summarizes abstracts in single sentences, organizes data from papers into tidy tables, and even lets you upload your own PDFs for analysis.
Working on a systematic review? Rayyan has become the researcher’s best friend. With over 500,000 users across 180+ countries, this clever tool learns from your screening decisions to make the process increasingly efficient.
Visual thinkers will love tools like Litmaps, Research Rabbit, and Connected Papers that create beautiful visualizations of relationships between studies. These maps help spot research gaps and promising new directions that might otherwise remain hidden.
The time savings are substantial – AI-assisted literature reviews typically take half the time of traditional methods, with equal or better quality. That means more hours for actual experiments rather than drowning in papers.
Working with a tight budget? Check out our AI Tools Online Free resource for cost-effective options.
AI tools for medical research in clinical trials & data analysis
Clinical trials – the most critical and costly phase of medical research. Did you know that half of the time and money spent bringing a new drug to market goes to clinical trials? And only one in seven drugs that enter phase I trials eventually gets approved.
Deep 6 AI is tackling the most time-consuming part of trial setup: patient recruitment. Using natural language processing on unstructured medical records, this platform can identify eligible patients in minutes instead of months. It analyzes physician notes, pathology reports, and genomic data to match patients to trials based on complex eligibility criteria – sometimes flagging potential candidates right at the moment of diagnosis.
Michael Chiang, Director of the National Eye Institute, notes: “The application of AI to clinical trials has the potential to dramatically reduce the time and cost of bringing new treatments to patients.”
For comprehensive trial management, Saama Technologies offers an end-to-end platform with AI that handles data cleaning, real-time monitoring, risk-based quality management, and even predicts trial outcomes.
Meanwhile, Medidata Solutions helps optimize trial design before you even begin. By analyzing historical trial data, their AI can predict enrollment rates, optimize site selection, and suggest protocol amendments that might improve success rates.
These tools are actively fighting “Eroom’s Law” – the troubling trend of declining returns on pharmaceutical R&D investment. By addressing key inefficiencies, AI tools for medical research are helping reverse this trend.
Recent research published in Nature Reviews Drug Findy has extensively documented this declining R&D productivity phenomenon. You can learn more about this research at https://doi.org/10.1038%2Fnrd3681.
Need to boost your analytical capabilities? Our AI Tools for Analytics guide offers additional resources and practical implementation strategies.
Imaging, Diagnostics & Personalized Treatment
Medical imaging was AI’s first major success story in healthcare, and today’s tools have reached or exceeded human-level performance in many diagnostic tasks.
Google Health AI has developed TB screening systems that match radiologists’ performance. Their ambitious initiative aims to provide 3 million free AI-powered screenings for TB, lung cancer, and breast cancer in India over the next decade – a critical intervention where TB causes over 1.3 million deaths annually.
For breast cancer detection, Northwestern Medicine’s collaboration with Google integrates AI directly into mammography workflows. Research confirms these AI systems match trained radiologists in detection capabilities – potentially saving countless lives through earlier diagnosis.
MedLM, a family of healthcare-tuned language models, supports clinical decisions by answering complex medical questions, summarizing patient histories, and extracting insights from unstructured medical data.
In the personalized medicine field, platforms like Causaly are revolutionizing how researchers understand disease mechanisms. Their knowledge graph contains over 500 million relationships between biological entities, helping researchers identify drug targets, find novel biomarkers, and analyze genetic data for truly personalized treatment planning.
The precision of these tools is enabling a shift toward medicine custom to individual patients based on their specific genetic makeup, biomarkers, and clinical history.
Automating Admin, Collaboration & Education
Let’s be honest – paperwork is the least exciting part of research. Administrative tasks eat up precious hours that could be spent on actual science. Thankfully, AI tools for medical research are taking on this burden too.
Medwise.ai serves as a next-generation search platform specifically designed for clinicians. It provides quick answers to clinical questions at the point of care, combining national knowledge resources with local guidelines. Researchers and clinicians can access evidence-based information within seconds, adopt local best practices, and drastically reduce time spent on manual searches.
For team collaboration, Labarchives offers an AI-improved electronic lab notebook that makes coordination and data sharing seamless while maintaining research integrity.
Virtual assistants are changing both medical education and patient interaction. Medical Chat helps doctors with daily work and patient education, while advanced simulation platforms create realistic training environments for students and researchers.
Behind the scenes, Natural Language Processing (NLP) tools are revolutionizing health record management by extracting key information from clinical notes, automating coding and billing, identifying potential research subjects, and generating structured data ready for analysis.
As one researcher aptly put it: “AI can’t compete at this point with human intelligence and compassion, but it can certainly free us from the administrative burden that takes away from our core scientific work.”
The best part? These tools don’t just save time – they create space for the human elements of research that truly matter: creativity, insight, and the passion to solve medical mysteries that could save millions of lives.
How to Integrate AI Tools into Your Medical Research Project
Let’s face it – implementing AI tools for medical research isn’t just about picking the shiniest new tech. It’s about thoughtfully integrating these tools into your existing workflow to make meaningful improvements. I’ve helped dozens of research teams through this process, and I’ve developed a practical roadmap that works consistently.
Step 1 – Define Objectives & Map the Research Workflow
Before diving into the AI toolbox, take a moment to identify exactly what you’re trying to accomplish. Think of this as drawing your map before starting the journey.
Start by having an honest conversation with your team about pain points. Where are you spending too much time for too little return? For many research teams, literature review consumes weeks of valuable time that could be better spent designing experiments or analyzing results.
Dr. Torben Riise, who incorporates Elicit into his daily research routine, shared a valuable insight with me: “Map out your entire research workflow first, then identify the specific stages where AI can have the most immediate impact.” This advice has saved countless hours for teams I’ve worked with.
Creating a simple workflow diagram can be eye-opening. When you visualize each step from initial literature review through data collection to publication, the bottlenecks often become obvious. One team I worked with finded they were spending nearly 40% of their time on administrative documentation – a perfect candidate for AI automation.
Getting stakeholder buy-in becomes much easier when you can point to concrete metrics. When presenting to administrators or funding bodies, highlight that teams using tools like Elicit have achieved a 50% reduction in time and cost for data extraction tasks. Numbers speak volumes when you’re making the case for investment.
Step 2 – Evaluate Compliance, Data Quality & Ethical Guardrails
Medical research operates in a highly regulated environment, and your AI implementation needs to respect these boundaries. This isn’t just about checking boxes – it’s about protecting patients and maintaining research integrity.
Start with a thorough compliance review. Any AI tools for medical research touching protected health information must be HIPAA compliant. For international collaborations, consider GDPR requirements. If your tools might influence clinical decisions, FDA regulations come into play. And don’t forget IRB approval for human subjects research.
The quality of your data directly impacts AI performance. I’ve seen brilliant AI implementations fail simply because the underlying data wasn’t ready. Take time to assess your data for completeness, standardization, and potential biases. One researcher I worked with finded their historical data contained significant gender imbalances that would have skewed their AI analysis if not addressed.
Ethical considerations should never be an afterthought. As one thoughtful researcher told me, “Presenting data alone is insufficient without humane and ethical context.” Develop clear protocols for informed consent, bias mitigation, and maintaining human oversight of AI systems.
Dr. Joel Chan, who regularly uses AI for literature exploration, offered this wise caution: “Always validate AI-generated outputs with human supervision to ensure accuracy and credibility.” The best implementations maintain this human-AI partnership rather than attempting full automation.
Step 3 – Select, Test & Deploy the Right AI Stack
With your objectives clear and compliance considerations addressed, you’re ready to choose the right tools for your specific needs. This is where the fun begins!
When evaluating AI tools for medical research, look beyond flashy features to assess alignment with your specific objectives. Consider compatibility with your existing systems, evidence of validation in peer-reviewed settings, and scalability as your research grows. User-friendliness matters too – even the most powerful tool won’t help if your team refuses to use it.
Start small with pilot projects. I’ve guided many teams through this process, and the most successful implementations begin with controlled testing. This approach lets you validate performance in your specific context and identify integration challenges early, before they become expensive problems.
Sandbox testing is particularly important when sensitive data is involved. Create a secure environment with anonymized data to test performance thoroughly without risking privacy breaches. One research team I worked with created a synthetic dataset that mimicked their actual patient data, allowing them to test their AI tools extensively before touching real patient information.
Don’t forget to consider scalability. Tools that work beautifully in a pilot may struggle when deployed across larger initiatives. Will the system handle larger datasets? Can it accommodate team expansion? Will it integrate with additional systems as needed? These questions can save you from painful limitations down the road.
For teams looking to build internal AI expertise, our Best Free Online Machine Learning Courses provide an excellent starting point. I’ve seen research teams transform their capabilities by investing in their own AI literacy.
Step 4 – Monitor Performance & Retrain Models
Implementing AI isn’t a “set it and forget it” proposition. It requires ongoing attention to ensure continued performance and value.
Establish clear key performance indicators that matter to your research. These might include time saved on routine tasks, accuracy compared to manual methods, or increases in publication output. One clinical trials team I worked with tracked patient recruitment velocity as their primary KPI, celebrating when their AI-assisted process reduced recruitment time by 62%.
Be mindful of model drift – the tendency of AI models to become less accurate over time as research practices evolve. Schedule regular retraining sessions to keep your tools performing at their best. This is especially important in rapidly evolving fields where new evidence emerges frequently.
Create feedback loops between your researchers and your AI systems. The most successful implementations I’ve seen maintain this human-in-the-loop approach, continuously refining based on user experience. Simple surveys or regular check-in meetings can capture valuable insights that improve your AI implementation.
Consider implementing a collaboration dashboard to track AI performance across projects. These centralized monitoring systems make it easy to spot issues early and share best practices across teams. As Michael Nielsen, PhD, wisely observed: “The most successful AI implementations in research are those that establish clear feedback mechanisms between the researchers and the AI systems they’re using.”
By following these steps, you’ll be well on your way to a successful integration of AI tools for medical research that truly improves your team’s capabilities rather than simply adding technological complexity. The goal isn’t to replace researchers but to amplify their impact by removing barriers and accelerating findy.
Conclusion & Next Steps
The integration of AI tools for medical research represents a paradigm shift in how we approach scientific findy in healthcare. From accelerating literature reviews to optimizing clinical trials and enabling personalized medicine, AI is addressing longstanding inefficiencies in the research process.
The impact is already measurable: patient screening reduced from weeks to minutes, literature reviews completed in half the time, and diagnostic systems matching or exceeding human expert performance. These efficiencies translate directly to faster findies, reduced costs, and ultimately, more lives saved.
At CheatCodesLab, we’re committed to helping researchers steer this AI revolution. Our certified AI tools and content strategies are designed to ensure that medical researchers can leverage the full potential of artificial intelligence without getting lost in technical complexities.
As you consider implementing AI in your research workflow, remember these key takeaways:
- Start with a clear assessment of your research objectives and pain points
- Ensure all tools meet rigorous compliance and ethical standards
- Begin with pilot projects before scaling to full implementation
- Establish continuous monitoring and improvement processes
The future of medical research is being shaped by those who effectively harness AI capabilities today. As Elbert Hubbard wisely noted, “One machine can perform the work of 50 ordinary men. No machine can do the work of one extraordinary man.” The goal of AI in medical research is not to replace human creativity and insight, but to amplify it—freeing researchers to focus on the extraordinary thinking that drives breakthrough findies.
Ready to transform your medical research workflow with AI? Explore our Artificial Intelligence Certification programs and visit our technology resources to stay updated on the latest developments in AI for medical research.
By embracing these powerful tools today, we can accelerate the pace of medical findy and bring life-saving treatments to patients faster than ever before.